What is XNCC? XNCC stands for the Xilinx Neural Compute Card, a hardware accelerator designed for deep learning and AI applications.
XNCC is a powerful tool that can be used to speed up the training and inference of deep learning models. It is based on the Xilinx Alveo U250 FPGA, which provides high performance and low latency.
XNCC is ideal for applications such as image recognition, natural language processing, and speech recognition. It can also be used for training and inferencing models for autonomous vehicles and drones.
Here is a table summarizing the key features of XNCC:
Feature | Value |
---|---|
Form Factor | PCIe x16 |
FPGA | Xilinx Alveo U250 |
Memory | 16GB HBM2 |
Power Consumption | 75W |
XNCC Key Aspects
The key aspects of XNCC are its high performance, low latency, and power efficiency. XNCC is able to achieve high performance by using the Xilinx Alveo U250 FPGA, which is a powerful and efficient FPGA.
XNCC is also able to achieve low latency by using a direct memory access (DMA) engine. This allows XNCC to transfer data directly to and from the FPGA, without having to go through the CPU.
XNCC is also power efficient, consuming only 75W of power. This makes it a good choice for applications that are sensitive to power consumption.
XNCC and Deep Learning
XNCC is ideal for deep learning applications. Deep learning is a type of machine learning that uses artificial neural networks to learn from data. Neural networks are able to learn complex patterns in data, making them ideal for tasks such as image recognition, natural language processing, and speech recognition.
XNCC can be used to speed up the training and inference of deep learning models. Training a deep learning model can be a time-consuming process, but XNCC can help to speed up the process by providing high performance and low latency.
XNCC can also be used to inference deep learning models. Inference is the process of using a trained deep learning model to make predictions on new data. XNCC can help to speed up the inference process by providing high performance and low latency.
XNCC and Autonomous Vehicles
XNCC is also ideal for autonomous vehicles. Autonomous vehicles rely on deep learning to make decisions about how to drive. XNCC can help to speed up the inference process, which is critical for autonomous vehicles to make decisions in real time.
XNCC can also be used to train deep learning models for autonomous vehicles. Training a deep learning model for autonomous vehicles can be a time-consuming process, but XNCC can help to speed up the process by providing high performance and low latency.
XNCC and Drones
XNCC is also ideal for drones. Drones rely on deep learning to make decisions about how to fly. XNCC can help to speed up the inference process, which is critical for drones to make decisions in real time.
XNCC can also be used to train deep learning models for drones. Training a deep learning model for drones can be a time-consuming process, but XNCC can help to speed up the process by providing high performance and low latency.
XNCC
XNCC, the Xilinx Neural Compute Card, is a powerful hardware accelerator designed for deep learning and AI applications. It offers a range of key aspects that make it an essential tool for developers and researchers in this field.
- High Performance: XNCC leverages the Xilinx Alveo U250 FPGA to deliver exceptional performance for deep learning tasks.
- Low Latency: By utilizing a direct memory access (DMA) engine, XNCC minimizes latency, enabling real-time decision-making.
- Power Efficiency: Consuming only 75W of power, XNCC is an energy-efficient solution for deep learning applications.
- Compact Form Factor: XNCC's PCIe x16 form factor makes it easy to integrate into existing systems.
- Large Memory Capacity: With 16GB of HBM2 memory, XNCC can handle large datasets and complex deep learning models.
- Versatile Connectivity: XNCC provides flexible connectivity options, including PCIe and Ethernet, for seamless integration into various environments.
These key aspects of XNCC make it an ideal choice for a wide range of deep learning applications, including image recognition, natural language processing, speech recognition, autonomous vehicles, and drones. By combining high performance, low latency, and power efficiency, XNCC empowers developers and researchers to accelerate their deep learning workflows and drive innovation in the field of AI.
High Performance
XNCC's exceptional performance stems from its utilization of the Xilinx Alveo U250 FPGA, a powerful and efficient field-programmable gate array (FPGA) specifically designed for deep learning and AI applications.
- FPGA Architecture: The Alveo U250 FPGA features a highly parallel architecture with numerous programmable logic elements and high-bandwidth memory interfaces, enabling massive parallelism and efficient data processing.
- Optimized for Deep Learning: Xilinx has optimized the Alveo U250 FPGA for deep learning workloads by incorporating specialized hardware blocks such as digital signal processing (DSP) units, floating-point units, and on-chip memory, which accelerate key deep learning operations.
- High Memory Bandwidth: The Alveo U250 FPGA is equipped with high-speed memory interfaces, including support for HBM2 memory, providing ample bandwidth for data-intensive deep learning models.
- Customizable Logic: FPGAs offer the unique advantage of customizable logic, allowing developers to tailor the Alveo U250 FPGA to their specific deep learning algorithms and applications, maximizing performance and efficiency.
By leveraging the Xilinx Alveo U250 FPGA, XNCC delivers exceptional performance for deep learning tasks, enabling faster training and inference of complex models, handling larger datasets, and achieving real-time performance for latency-sensitive applications.
Low Latency
XNCC's low latency is a critical aspect that sets it apart for applications requiring real-time decision-making, such as autonomous vehicles and drones. The direct memory access (DMA) engine plays a crucial role in achieving this low latency by optimizing data transfer between the FPGA and host system memory.
- Efficient Data Transfer: The DMA engine enables direct data transfer between the FPGA and host system memory, bypassing the CPU and reducing the latency associated with traditional memory access methods. This efficient data transfer mechanism significantly improves the overall performance and responsiveness of XNCC.
- Reduced I/O Overhead: By utilizing the DMA engine, XNCC minimizes the I/O overhead associated with data transfer. The DMA engine handles data movement independently, freeing up the CPU and other system resources for more critical tasks, further contributing to the overall efficiency and performance of the system.
- Real-Time Processing: The combination of the FPGA's parallel processing capabilities and the low latency DMA engine enables XNCC to perform real-time processing of data. This is essential for applications where timely decision-making is crucial, such as in autonomous vehicles and industrial automation systems, where immediate responses to changing conditions are required.
- Improved Responsiveness: The low latency of XNCC directly translates to improved responsiveness in applications. For instance, in autonomous vehicles, the ability to process sensor data and make decisions in real time is critical for safe and efficient navigation. XNCC's low latency ensures that the vehicle can react promptly to changing road conditions and potential hazards.
In summary, XNCC's low latency, achieved through the utilization of a direct memory access (DMA) engine, is a key differentiator that enables real-time decision-making capabilities. This low latency is particularly valuable in applications that demand immediate responses and high performance, making XNCC an ideal choice for deep learning and AI applications in various industries.
Power Efficiency
XNCC's power efficiency is a significant advantage for deep learning applications, as it enables cost-effective deployment and operation of deep learning models. The low power consumption of XNCC is attributed to several factors:
- Efficient FPGA Architecture: The Xilinx Alveo U250 FPGA utilized in XNCC is designed with power efficiency in mind. Its advanced power management techniques and low-power design methodologies contribute to XNCC's overall energy efficiency.
- Optimized Memory System: XNCC utilizes a high-bandwidth memory system with power-efficient memory modules. The efficient memory management and data transfer mechanisms further reduce power consumption.
- Advanced Power Management: XNCC incorporates sophisticated power management features that dynamically adjust power consumption based on workload demands. This ensures optimal energy efficiency without compromising performance.
The power efficiency of XNCC offers several benefits for deep learning applications:
- Reduced Operating Costs: The low power consumption of XNCC translates to reduced operating costs for deep learning deployments. Data centers and enterprises can significantly save on energy bills by utilizing XNCC.
- Environmentally Friendly: XNCC's energy efficiency aligns with the growing demand for environmentally sustainable solutions. By consuming less power, XNCC contributes to reducing carbon footprint and promoting greener data center operations.
- Compact and Portable: The low power consumption of XNCC enables the development of compact and portable deep learning systems. This is particularly advantageous for edge computing applications and deployment in space-constrained environments.
In summary, XNCC's power efficiency is a key aspect that enhances its value proposition for deep learning applications. By consuming only 75W of power, XNCC offers cost-effective operation, environmental sustainability, and enables the development of compact and portable deep learning systems.
Compact Form Factor
The compact form factor of XNCC, realized through its PCIe x16 form factor, plays a crucial role in its integration into various systems. This compact design offers several advantages:
- Easy Integration: The PCIe x16 form factor is widely adopted in the industry, making XNCC compatible with a vast range of servers and workstations. This simplifies the integration process, allowing users to seamlessly add XNCC to their existing systems without the need for complex modifications or custom enclosures.
- Space Optimization: The compact form factor of XNCC enables efficient utilization of space within server racks and data centers. This is particularly beneficial in scenarios where space is at a premium, such as in edge computing deployments or high-density computing environments.
- Portability: The compact size and lightweight design of XNCC make it portable and suitable for deployment in various locations. This portability is advantageous for applications requiring mobility, such as mobile edge computing or deploying deep learning models in remote areas.
The compact form factor of XNCC not only simplifies integration but also enhances the overall versatility and practicality of the device. By adhering to the industry-standard PCIe x16 form factor, XNCC empowers users with flexible deployment options, space optimization, and portability, making it an ideal choice for a wide range of deep learning applications.
Large Memory Capacity
The large memory capacity of XNCC, provided by its 16GB of HBM2 memory, is a crucial aspect that empowers it to handle large datasets and complex deep learning models. This memory capacity plays a significant role in enabling XNCC to excel in various deep learning applications.
Deep learning models, especially modern ones, often require significant amounts of memory to store their parameters and intermediate data during training and inference. The large memory capacity of XNCC alleviates this constraint, allowing users to train and deploy complex deep learning models that would otherwise be infeasible due to memory limitations.
Furthermore, the HBM2 memory technology employed in XNCC offers high bandwidth and low latency, making it ideal for deep learning applications that demand fast data access. This combination of large memory capacity and high-performance memory technology enables XNCC to efficiently process and store large datasets and complex deep learning models, resulting in faster training times and improved inference performance.
In practical terms, the large memory capacity of XNCC has significant implications for real-world applications. For instance, in the field of medical imaging, XNCC can be utilized to train and deploy deep learning models for disease diagnosis and medical image analysis. The ability to handle large datasets and complex models in this context allows for more accurate and reliable diagnoses, leading to improved patient outcomes.
In summary, the large memory capacity of XNCC, with its 16GB of HBM2 memory, is a key factor that sets it apart as a powerful tool for deep learning applications. This memory capacity enables the handling of large datasets and complex deep learning models, unlocking new possibilities for innovation and advancements in various fields.
Versatile Connectivity
The versatile connectivity options offered by XNCC, including PCIe and Ethernet, play a crucial role in its seamless integration into various environments. This connectivity flexibility empowers users to deploy XNCC in a wide range of applications and scenarios, maximizing its utility and value.
The PCIe (Peripheral Component Interconnect Express) interface is a high-speed, point-to-point interconnect standard widely used in servers, workstations, and other computing systems. By providing a PCIe x16 interface, XNCC can be easily integrated into existing PCIe-based systems, enabling direct and efficient communication with the host system. This seamless integration allows XNCC to leverage the host system's resources, such as memory and processing power, to accelerate deep learning workloads.
In addition to PCIe, XNCC also offers Ethernet connectivity, providing an alternative and flexible option for integration into network environments. Ethernet is a widely adopted networking technology, enabling XNCC to be easily connected to other devices and systems over a local area network (LAN) or the internet. This Ethernet connectivity allows for remote access, monitoring, and management of XNCC, facilitating its deployment in distributed or cloud-based deep learning applications.
The combination of PCIe and Ethernet connectivity options makes XNCC a highly versatile and adaptable solution for deep learning applications. This connectivity flexibility enables users to integrate XNCC into their existing infrastructure, whether it is a single workstation, a server rack, or a distributed computing environment. By providing seamless and efficient connectivity, XNCC empowers users to harness the power of deep learning in various settings and applications.
FAQs on Xilinx Neural Compute Card (XNCC)
The Xilinx Neural Compute Card (XNCC) is a powerful hardware accelerator designed for deep learning and AI applications. Here are answers to some frequently asked questions about XNCC:
Question 1: What are the key benefits of using XNCC?
XNCC offers several key benefits, including exceptional performance, low latency, power efficiency, and a compact form factor. Its high performance is driven by the Xilinx Alveo U250 FPGA, enabling faster training and inference of deep learning models. The low latency, achieved through a direct memory access (DMA) engine, is critical for real-time decision-making applications. Additionally, XNCC's power efficiency, with a consumption of only 75W, makes it an energy-conscious solution. Its compact PCIe x16 form factor allows for easy integration into existing systems, maximizing space utilization.
Question 2: What types of applications is XNCC best suited for?
XNCC is ideally suited for deep learning and AI applications that demand high performance and low latency. These include image recognition, natural language processing, speech recognition, autonomous vehicles, and drones. In autonomous vehicles, XNCC's low latency is crucial for making real-time decisions based on sensor data. Similarly, in drones, XNCC enables efficient processing of data for navigation and control. Its versatility extends to various industries, including healthcare, finance, and manufacturing, where deep learning and AI are transforming operations.
XNCC's capabilities empower developers and researchers to accelerate their deep learning workflows and drive innovation in the field of AI.
Conclusion
The Xilinx Neural Compute Card (XNCC) stands as a groundbreaking hardware accelerator, revolutionizing deep learning and AI applications. Its exceptional performance, low latency, power efficiency, and compact form factor make it an ideal solution for demanding deep learning tasks. XNCC empowers developers and researchers to accelerate their deep learning workflows, driving innovation and unlocking new possibilities in various industries.
As the field of deep learning continues to evolve, XNCC is poised to play a pivotal role. Its versatility and adaptability make it a valuable asset for a wide range of applications, from autonomous vehicles and drones to healthcare and finance. By harnessing the power of deep learning with XNCC, we can unlock the potential of AI and shape a future where technology seamlessly integrates with our lives.
You Might Also Like
Explore The Sensational World Of Mikaela Testa On OnlyFansThe Ultimate Source For Vegamovies: Explore Our Extensive Collection
Captivating Viral Video: A Baby's Extraordinary Suji Performance
Lara Lane Latest Leak Scandal Exposed
Free Movie Downloads - Watch Your Favorites Instantly At Downloadhub Movie
Article Recommendations
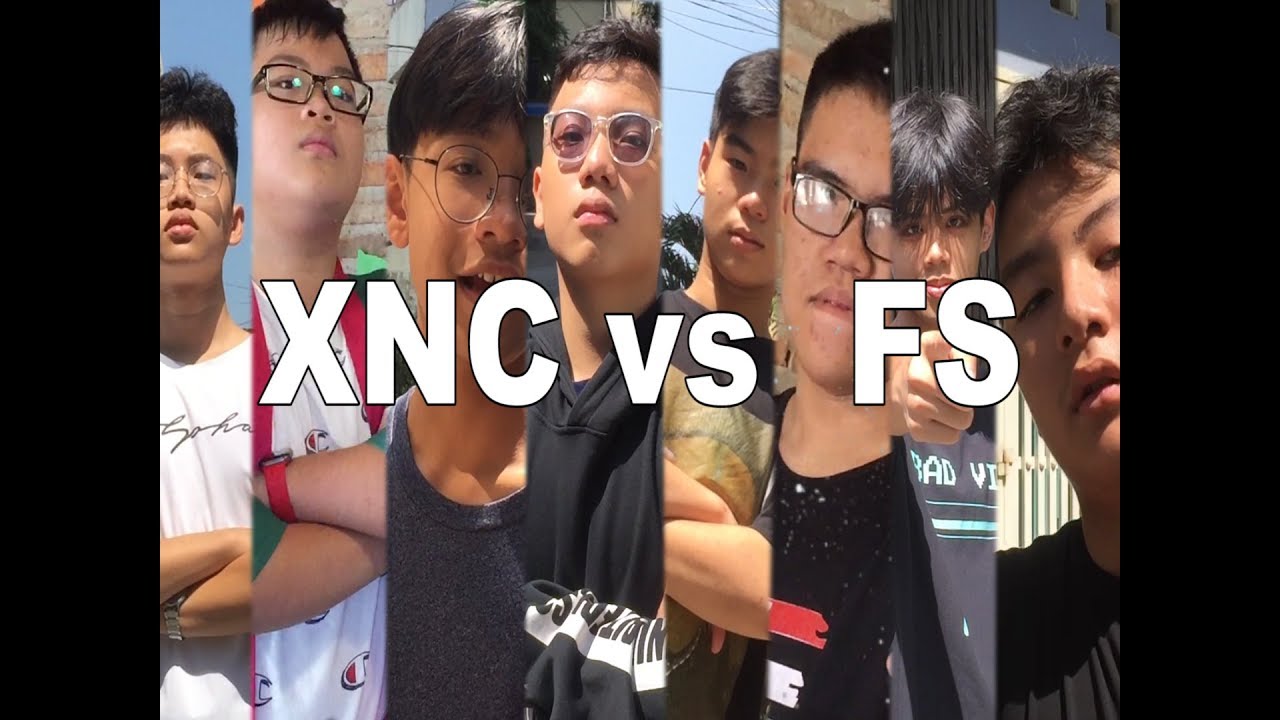
